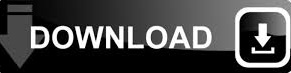


For the pattern analysis tools, it is the probability that the observed spatial pattern was created by some random process. Whenever you see spatial structure such as clustering in the landscape (or in your spatial data), you are seeing evidence of some underlying spatial processes at work, and as a geographer or GIS analyst, this is often what you are most interested in. Often, you will run one of the pattern analysis tools, hoping that the z-score and p-value will indicate that you can reject the null hypothesis, because it would indicate that rather than a random pattern, your features (or the values associated with your features) exhibit statistically significant clustering or dispersion. The z-scores and p-values returned by the pattern analysis tools tell you whether you can reject that null hypothesis or not. The null hypothesis for the pattern analysis tools ( Analyzing Patterns toolset and Mapping Clusters toolset) is Complete Spatial Randomness (CSR), either of the features themselves or of the values associated with those features.

Most statistical tests begin by identifying a null hypothesis.

Such events may be considered as very unlikely: accidents and mishaps, on the one hand, and streaks of luck, on the other. If this principle is successfully applied you can expect to have 3.4 defects for every one million realizations of a process. If you try to expand this interval and go six sigmas to left and right, you will find out that 99.9999998027% of your data points fall into this principles. Hence, only 0.03% of all the possible realizations of this process will lay outside of the three sigma interval. 99.7% of observation of a process that follows the normal distribution can be found either to the right or to the left from the distribution mean.
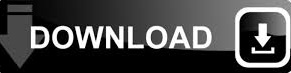